Abstract:
Plant height (PH) is an essential trait in maize (Zea mays) that is tightly associated with planting density, biomass, lodging resistance, and grain yield in the field. Dissecting the dynamics of maize plant architec-ture will be beneficial for ideotype-based maize breeding and prediction, as the genetic basis controlling PH in maize remains largely unknown. In this study, we developed an automated high-throughput pheno-typing platform (HTP) to systematically and noninvasively quantify 77 image-based traits (i-traits) and 20 field traits (f-traits) for 228 maize inbred lines across all developmental stages. Time-resolved i-traits with novel digital phenotypes and complex correlations with agronomic traits were characterized to reveal the dynamics of maize growth. An i-trait-based genome-wide association study identified 4945 trait-associated SNPs, 2603 genetic loci, and 1974 corresponding candidate genes. We found that rapid growth of maize plants occurs mainly at two developmental stages, stage 2 (S2) to S3 and S5 to S6, accounting for the final PH indicators. By integrating the PH-association network with the transcriptome profiles of spe-cific internodes, we revealed 13 hub genes that may play vital roles during rapid growth. The candidate genes and novel i-traits identified at multiple growth stages may be used as potential indicators for final PH in maize. One candidate gene, ZmVATE, was functionally validated and shown to regulate PH-related traits in maize using genetic mutation. Furthermore, machine learning was used to build predictive models for final PH based on i-traits, and their performance was assessed across developmental stages. Moderate, strong, and very strong correlations between predictions and experimental datasets were achieved from the early S4 (tenth-leaf) stage. Colletively, our study provides a valuable tool for dissecting the spatiotem-poral formation of specific internodes and the genetic architecture of PH, as well as resources and predic-tive models that are useful for molecular design breeding and predicting maize varieties with ideal plant architectures.
KeyWords:
phenomics;;GWAS;;plant height;;machine learning;;prediction;;maize
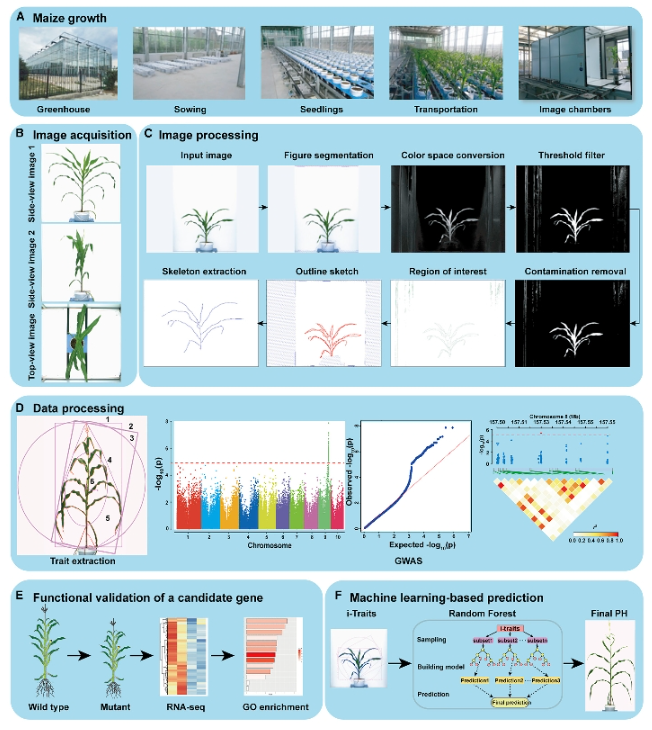 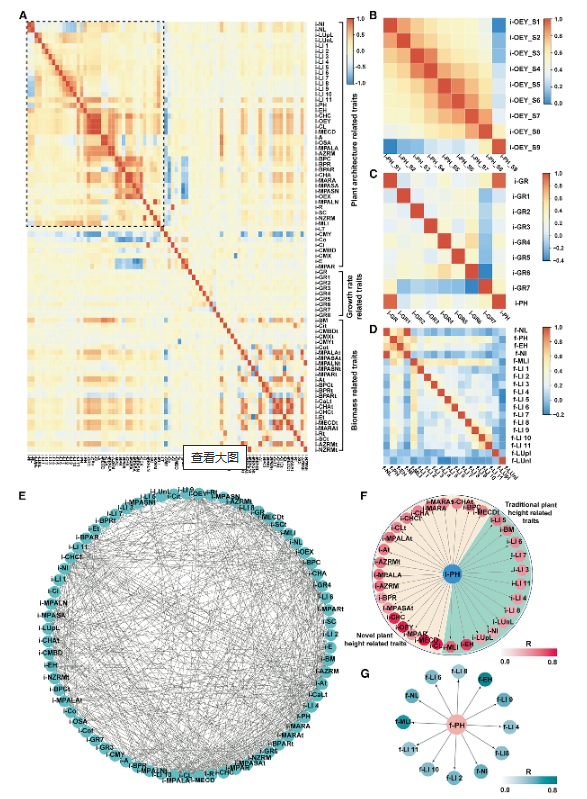
|